The early days of a subscription business are exciting as you get your first signups and the payments roll in from happy customers. At some point though, you’ll start to encounter payments that fail for one reason or another. You'll try to re-process them, some with success, some without.
As your business grows, so will your failed payments — and the reasons for failed payments will be harder to manage. For example, more customers means more card issuers interacting with your payment processor, each with its own set of authentication requirements to approve a payment. This complexity makes it nearly impossible to develop a set of rules to guarantee the highest success for payment processing.
But machine learning doesn't play by a single set of rules. Instead, it can address the unique combination of payment processing factors in a way that traditional batch processing simply can’t.
The Role of Machine Learning in the Payments Industry
Machine learning has revolutionized the payments industry, addressing key issues for the many players in the transaction, from the consumer to the merchant to the issuing banks and card networks. Rather than follow a linear path for payment authorization, processing, settlement, and retries and recoveries, machine learning can handle a complex set of scenarios, applying different logic at the transaction level.
According to Cardinal Commerce, false declines are more than $400 billion — a significant amount of lost revenue. It’s a problem that continues to grow, even as companies work to address the root causes. Machine learning can help with recovering failed payments by identifying the optimal time and data needed to re-process a transaction. This improves the success rate and streamlines the recovery process.
While payment authorization and processing happen in the background, ML technology can reach the forefront of the customer experience. The customer can be provided with personalized offers or recommendations using transaction data.
In all of these instances, machine learning is only as good as its training data. It should be developed by industry experts and is most effective with access to large datasets. The more data available, the more the technology can “learn” and improve.
4 Proven Ways for Recurring Payments Recovery
As the customer base grows and subscription businesses grapple with more failed payments, they’ll need to develop a plan for payments recovery.
Remember that the customer didn’t intend for a payment to fail, and the failed payment is now a source of friction in the subscription payment process. Without effective recovery tactics, the customer will churn, and the recurring revenue will be lost. There are four types of recovery tactics:
- Customer Engagement: Client communications offer convenience but risk over-investment in impersonal messaging, potentially inconveniencing customers and undermining their subscription experience.
- Internal Payments Team: Optimizing core product development often takes precedence over building complex internal payment recovery teams due to the dynamic and resource-intensive nature of addressing evolving failed payment challenges, which can especially burden smaller companies with limited resources.
- External Vendors/Tools: Companies can outsource payment recoveries to a third party to manage. While this alleviates internal teams, many outsourced vendors rely on reprocessing payments in batches, which doesn’t account for the nuanced reasons a payment can fail.
- Machine Learning Technology: Leveraging artificial intelligence and machine learning in payment recovery optimizes for diverse factors, allowing individualized and efficient approaches to collecting payments, overcoming limitations of static methods and achieving quicker revenue recovery.
Customers will only go so far to correct the issue themselves and feel inconvenienced if the payment failure was due to a behind-the-scenes processing issue. The more you can improve your failed payments recovery, the better for your business.
How Machine Learning Can Maximize Recurring Payments Recovery
Unlike other methods for recovering failed payments, machine learning can pull at different levers simultaneously. As additional data is ingested, the technology “learns” and improves, resulting in more successful payment recoveries.
Rules-based systems don’t work because they are limited by a series of “if this failure happens, try this type of recovery.” They follow the same sequence of events, unable to account for the nuances of the specific payment failure. This tactic will recover some failed payments, but still leave others on the table. Machine learning doesn’t have the same limitations and learns in real-time, every day.
Understanding the Right Data
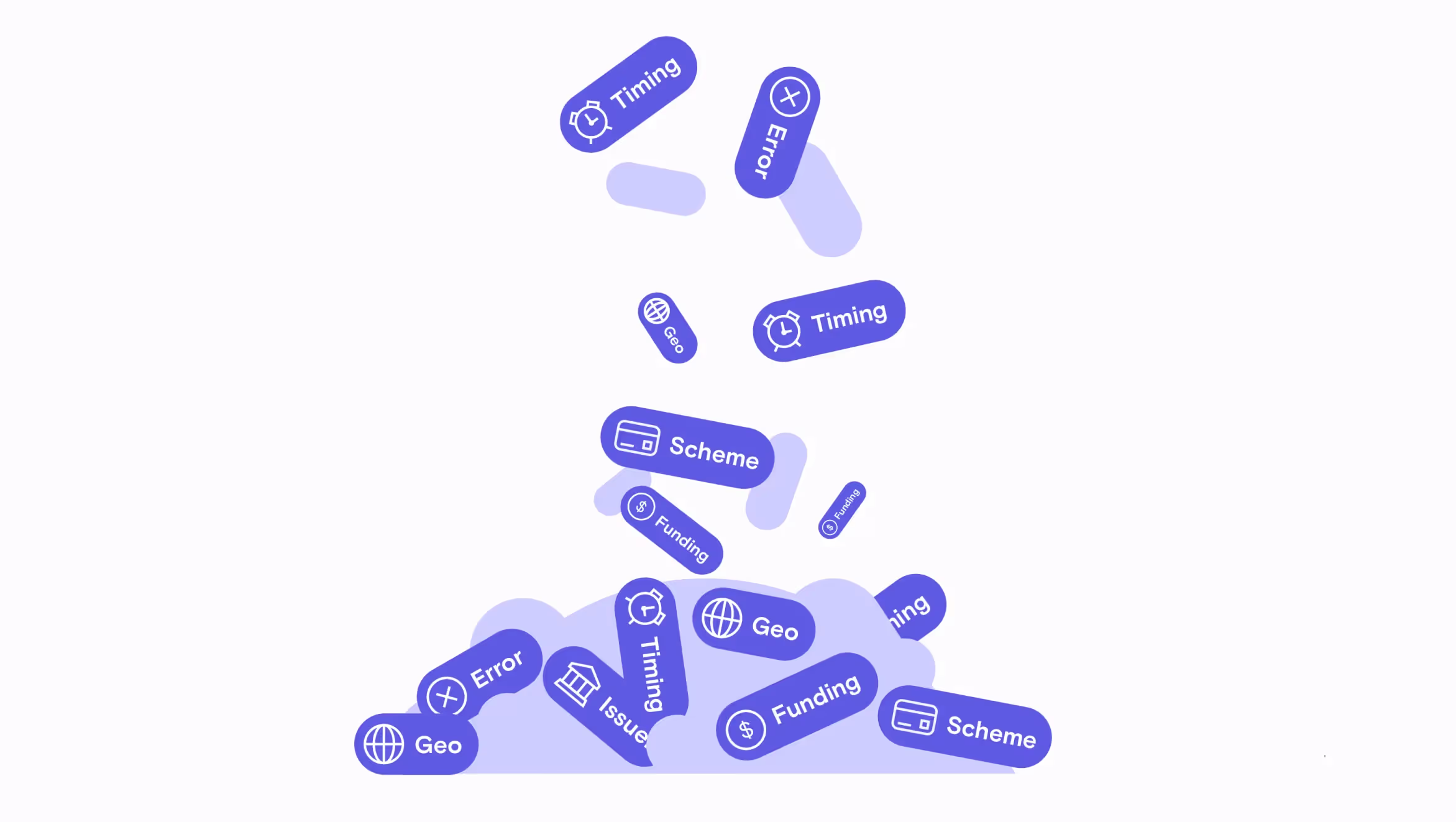
The data required for each transaction is complicated because it varies by issuing bank, card network, and the regulatory requirements of each country — any of which can change without warning.
Machine learning analyzes more than a hundred possible transaction data points sent on the payload to the processor and adjusts based on failed payment data and error codes. While human analysts have to decipher error codes (which are often unclear or incorrect), machine learning can look for patterns in the data and the type of card. When transactions are successfully processed, the technology also learns from that and applies the findings to other transactions.
Optimizing Each Transaction
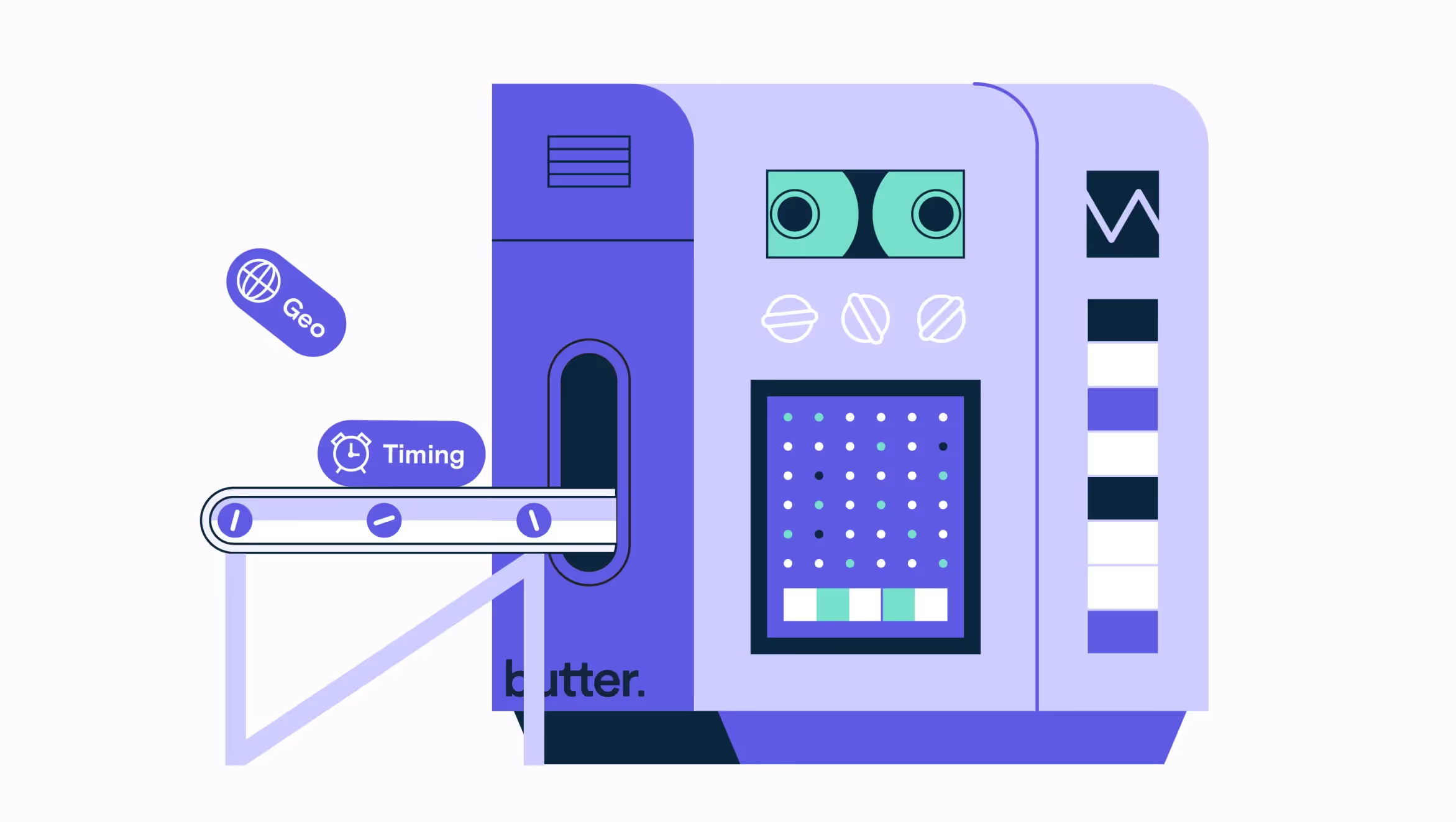
The ideal time to retry payment processing can vary depending on the customer’s location or expected availability of funds (such as a payroll deposit). However, payment retries are often done in batches, which re-processes the failed payments in bulk. Machine learning looks at the specific transaction and determines the optimal time to retry, optimizing recovery.
Some payments teams have used their own resources to develop ML technology. The payments team at Dropbox used their internal resources to develop machine learning technology to address failed payments. In Dropbox’s case, a failed payment meant that the customer would be downgraded from a paid plan to a free plan — an overall negative customer experience. The payments team had a theory that they could use predictive signals for each customer to improve payment retry outcomes. The ML team was successful with its approach and drove “outsized business and user value.”
As the team at Dropbox found, when retries are processed with a precise, timing-based approach, not only is the subscription business preserved, but the customer experience is improved. Customers won’t miss a product delivery or have a service interruption.
Optimizing the Number of Retries

Every payments team needs to be aware of its transaction authorization rate (TAR), or the percentage of transactions approved by a payment processor or financial institution. Ideally, this number should be between 80% to 90%. Issuing banks are more likely to deny an online transaction, even if it's legitimate, because of an increased risk of fraud. Merchants aren’t left with much recourse, other than to attempt the payment again.
As the merchant keeps trying to recover failed payments the bank is more likely to suspect fraud and start lowering the authorization rate. It becomes a vicious cycle: the more the merchant tries to retry failed payments the more failed payments it will get. Additional retries have other consequences as well, such as higher PSP fees and lower success with pre-authorizations sent to the customer’s bank.
Machine learning creates logic for each merchant by studying their payments history and combining it with each payment’s data, such as timing. As a result, the model can identify if a failed payment is likely to fail when resubmitted or if it’s worth retrying and optimizes the number of retries.
Recover Revenue Faster
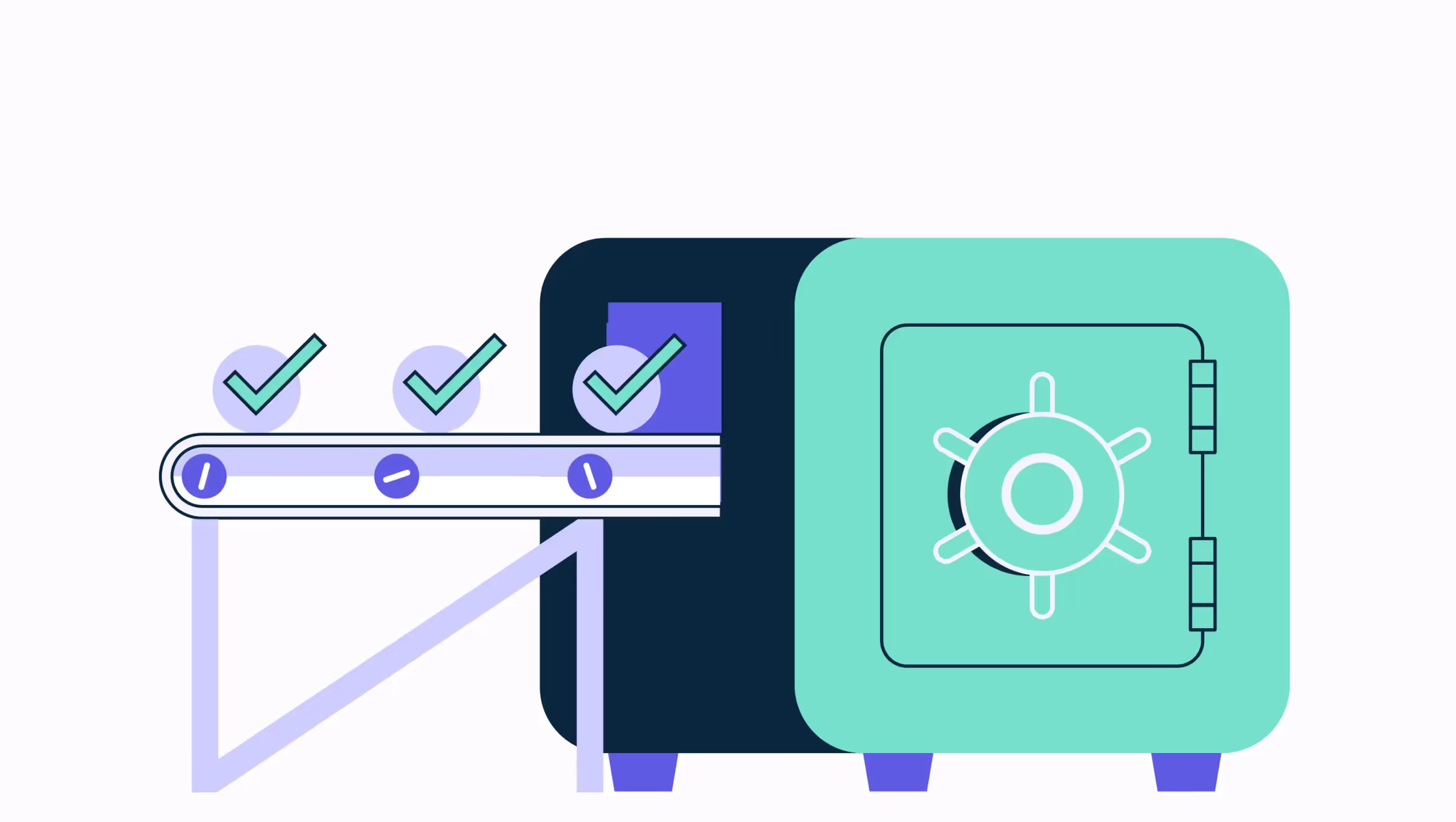
When failed payments are retried in batches, it can take time before a transaction is finally successful. Retries will happen at intervals, every few days, and it can take months (depending on the dunning cycle) before a payment finally goes through (if at all). Meanwhile, the customer’s account has been impacted. For companies shipping physical goods, this can also cause critical supply chain issues.
Machine learning, by contrast, takes days on average to recover a failed payment. Not only is this better for the customer, but also gets money into the business’s account more quickly.
Leverage the Power of Machine Learning to Increase Your Revenue
Machine learning evaluates each transaction against the thousands of reasons why a payment might fail, operating silently in the background to attempt payment recovery.
It’s the combination of data and timing where you’ll start to see exponential growth in payments recovery. Butter’s customers have seen anywhere from 20% to 200% increased lift in recovered payments with our machine learning technology. Across all customers, we’ve seen a 9% decrease in churn — subscribers that might have otherwise been lost.
Our technology also incorporates merchant-specific algorithms. We use data to determine what’s happening in the industry across the globe and how to optimize for a particular merchant. The customized model adapts to the nuances of your subscription model and customers.
Machine learning is the best way to solve the challenges of failed payments. Contact Butter for your free Payment Health Analysis.